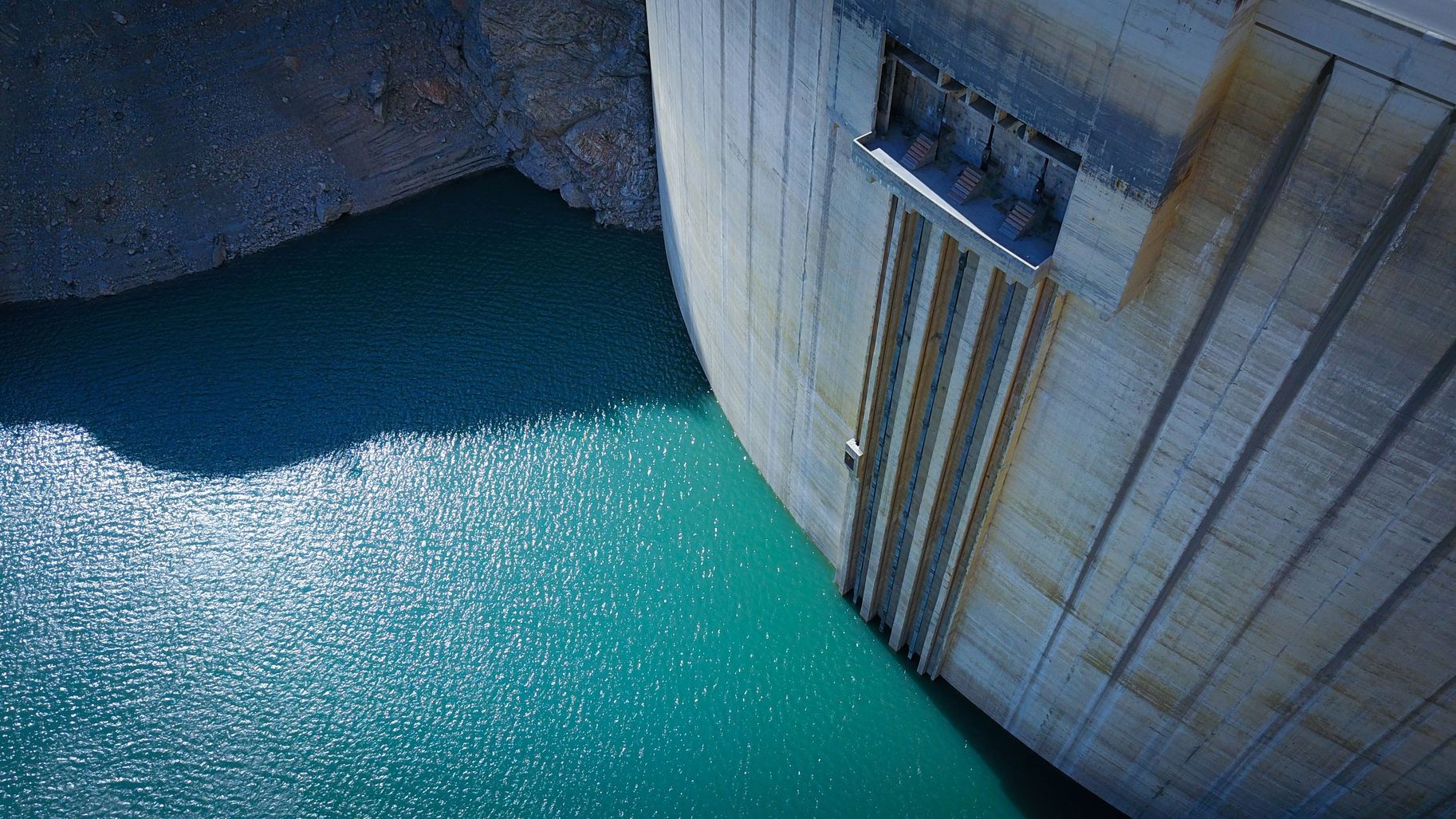
We all know the capacity of statistics to predict average behaviour: this is what enables the creatioResearchn of voting intention surveys using a representative sample of 1,000 people, or the prediction of average rainfall based on the meteorological data of past years. However, it is more difficult to predict - based on these same data - the scale of extreme events, such as 100-year floods i.e. the flooding of a river that only occurs once every 100 years. And yet this is what researchers from the Mistis team at the Inria centre in Grenoble - who co-financed a thesis on this subject with EDF R&D - are working on. “For EDF, a better prediction of these meteorological disasters is crucial in order to better size the resistance of structures such as hydraulic dams or nuclear plants”, Stéphane Girard, research director in the Mistis team, explains.
Extreme value theory
To this end EDF teams have, for around the past 10 years, been using a set of statistical methods originating from the "extreme value theory", put in place by Dutch mathematicians following the terrible tidal wave that submerged the North Sea coasts on 1 February 1953, causing more than 1,800 deaths. “Their aim was to better predict such disasters, even though we often only have very few - if any - examples of similar events at our disposal”, Stéphane Girard explains. For example, in the case of the Netherlands, the previous tidal wave of a comparable size took place in... 1570. “The advantage of the extreme value theory is that it makes it possible to estimate the probability of extreme events using a set of average data. The disadvantage is that the uncertainty linked to these extrapolations is even more significant if we want to predict over the long term, for example a 1,000-year - rather than a 100-year - flood. The whole purpose of our work is to quantify these uncertainties more accurately”, Clément Albert, author of the thesis, explains.
And also for autonomous cars
By basing himself on regional measurements of rainfall, river flow and wind speeds collected over more than 100 years, Clément Albert therefore endeavoured to quantify the reliability of EDF R&D's statistical tools. “For example, I looked to see if the predictions made based on the first years of measurements were proven by the last years of measurements, by comparing the model to the reality”, the researcher says. The conclusions of this thesis, completed in December 2018, will enable the EDF teams to know what level of confidence they can attribute to each statistical prediction, and to take this into account when sizing the structures. However, they could also benefit other strategic areas, such as autonomous cars. “Autonomous driving is based on sensors that constantly measure the distances between the car and obstacles. Manufacturers are doing everything they can to best characterise the reliability of these sensors. In this context our methods would, for example, enable an estimation of the length of the test drive phase that must be carried out in order to derive a reliable to-scale prediction of the lifespan of a vehicle”, Stéphane Girard explains.