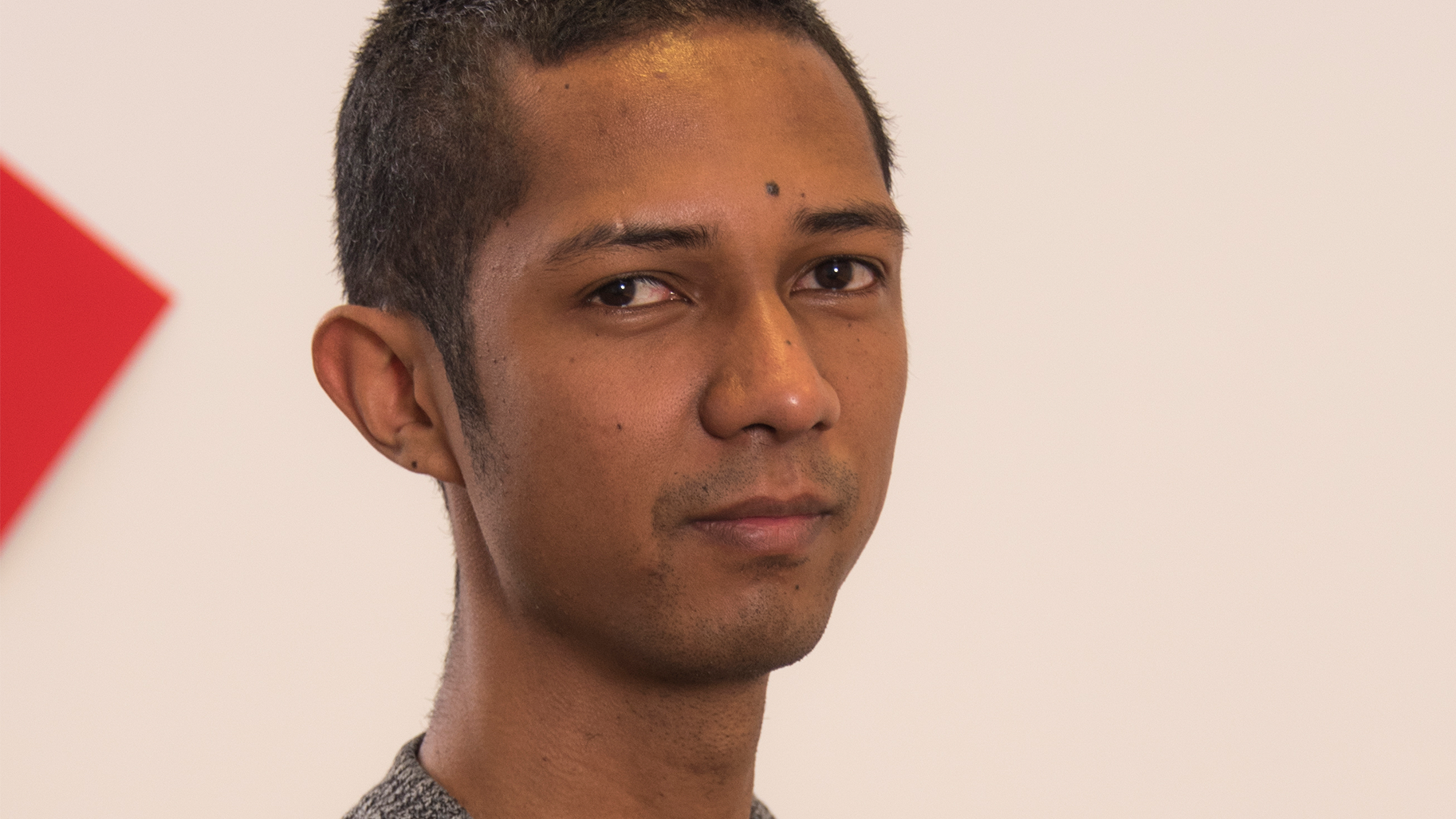
Embedded navigation systems for cars, email spam detection, medical imagery recognition and analysis, recommendation for an audiovisual platform, the steering of a lawnmower or robot vacuum cleaner, or the generation of news flows for a social network...these digital applications of our daily professional and private lives share a common feature: they are all driven by machine learning algorithms, which are to artificial intelligence what learning is to the human brain.
‘An ML model is developed in two phases, supervised by a data scientist’, says Herilalaina Rakotoarison to explain the implementation context of his thesis on the subject: Some contributions to AutoML: hyper-parameter opimization and meta-learning. ‘First you have to prepare the training data which will enable the ML model to learn to solve the problem it is designed to deal with, then you select the most appropriate algorithm for the aim, which will be executed on all this data, in addition to the hyper-parameters whose value is used to oversee the learning process. Experimented with according to an iterative process until a satisfactory result is obtained, the ML model can then be used on new data which the algorithm will process thanks to what it learnt.’
Machine learning applied to Machine learning
While, in practice, numerous software programmes and platforms enable data scientists to explore, test and produce an ML model, the difficulty lies in the choice of the latter.
There are a great deal of ML algorithms and a huge variety of possible values for the associated hyper-parameters. Choosing the most effective model in terms of the expected results is often a lengthy, complex and therefore costly task. The aim of AutoML is thus to facilitate these operations by automating them.
This means using an ML model to select and configure the ML model required.’ A sort of squared ML. Herilalaina Rakotoarison’s thesis, under the supervision of Marc Schoenauer and Michèle Sebag, co-directors of the TAU project-team (Inria, Université Paris-Saclay, CNRS within the LISN), presents two contributions aimed at improving AutoML methods by drawing on the two most researched aspects in this field: optimisation and meta-learning.
An algorithm increasingly close to humans
‘For the first contribution, the idea was to split the optimisation process in two and find a way to resolve the issues of structural optimisation, (i.e. the choice of algorithms), and parametric optimisation, (i.e. the configuration of hyper-parameters), using two combined strategies.’ The solution offered is a hybrid optimisation algorithm called Mosaic, which has a unique way of blending tree search methods and Bayesian optimisation, whose assessment demonstrates an empirical performance exceeding that of the AutoML systems usually applied.
‘The second aim of my thesis was to provide an algorithm which could learn similarities between tasks. The initial hypothesis is that two tasks can be considered as similar if the optimal algorithms to process them are the same.’
This ability of an algorithm to learn from past experience, which is described as meta-learning, opens up particularly interesting prospects because it operates on a higher level than that of traditional machine learning, its ultimate aim being to learn autonomously by adapting to a sequence of potentially linked tasks, much as a human being does.
Promising prospects
These prospects reach well beyond the sphere of fundamental research, as one of the aims behind AutoML is to enable users without specialised knowledge of artificial intelligence to build efficient ML specifically adapted to their sector, for example medicine or climate change.
While Herilalaina Rakotoarison’s work represents a significant advance in AutoML research, there are still many paths to explore in this area. This is the task he is now setting himself as part of a postdoctorate at the Machine Learning Lab of Freiburg University, ‘one of the world’s most advanced laboratories in the field of AutoML research.’