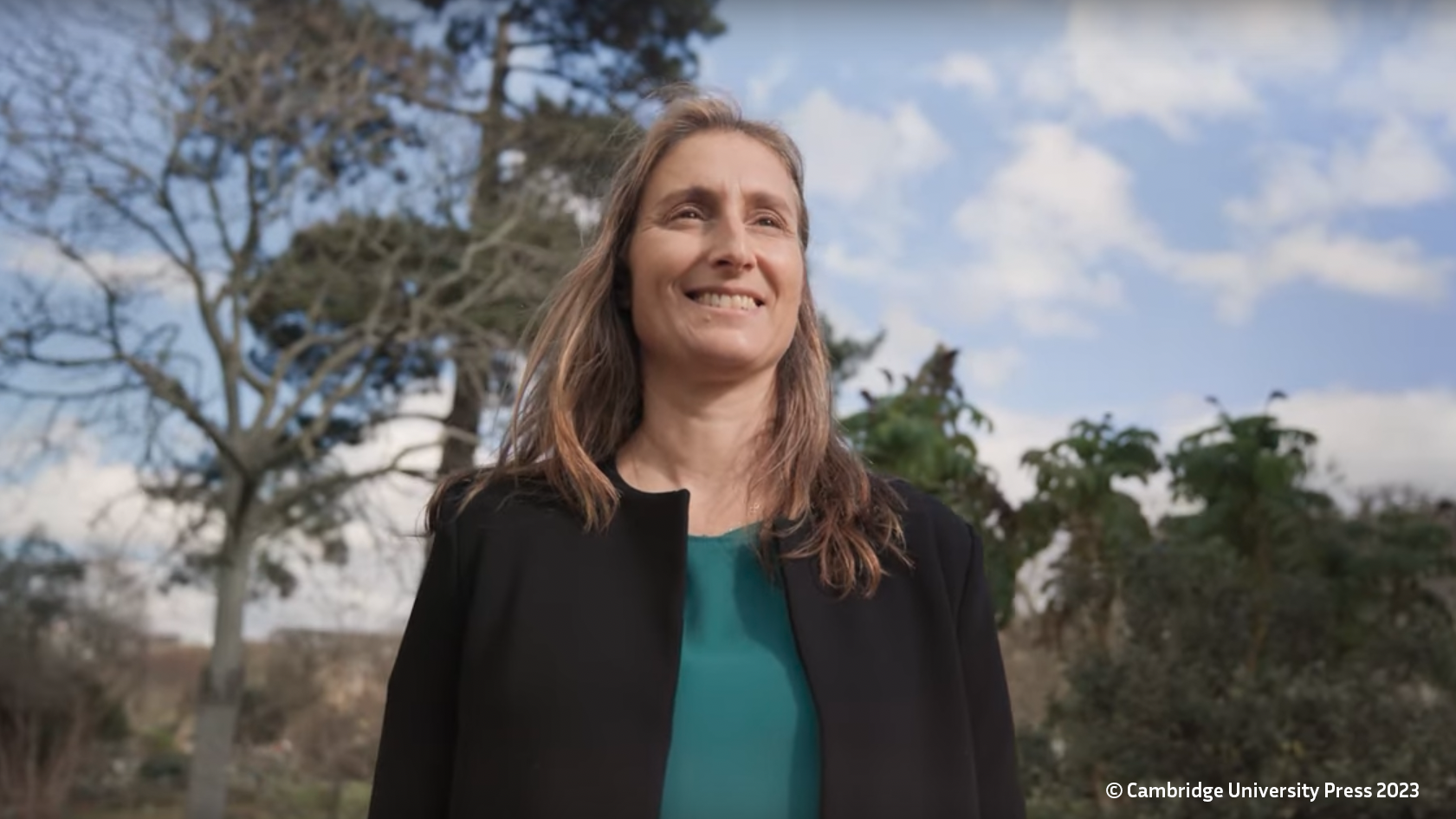
Climate science is an extremely data-rich field, as Claire Monteleoni reminds us:
Climate simulation models, which are based on physics, have generated a staggering amount of data. Some climate scientists estimate that there is more model-simulated data available than all earth-observing satellite data.
The challenge of big data in confronting climate change
The rise in the number of weather stations, radars, weather balloons and satellites means there is ever more information on changing water levels in the oceans, melting glaciers, the health of forests and changes to the composition of the atmosphere. Observation data also includes information that goes back thousands of years (what is known as paleoclimatology), based on analysis of natural archives such as ice cores, tree rings, coral, foraminifera and pollen.
Meanwhile, physics-driven climate models have been extremely useful in understanding and predicting climate change. Based on highly complex mathematical models, they take the form of large-scale computer simulations in which geophysicists, climatologists and meteorologists all “encode” their knowledge. In addition to being heterogeneous all of these (observed and simulated) data sets are variable in both time and space.
Despite the massive volumes of data, there is still a lot of uncertainty about climate change, and its short and long-term impacts. For example, forecasting sudden extreme weather events remains challenging, particularly at a local level.
Claire Monteleoni is well aware of the problems linked to big data. She came to the Inria Paris centre in January 2023 as part of the Choose France programme of the national AI strategy launched by President Macron in 2018. Claire Monteleoni's aim is to establish a new team of scientists in the field of artificial intelligence research for confronting climate change.
Aside from the unwieldy volumes of data, scientists have encountered other issues, including a lack of available data in certain parts of the world. As Claire Monteleoni explains, a lot of data is collected for certain regions, but for others it remains limited. Not only could AI help scientists to anticipate upcoming changes by filling in gaps in the data, but it could also provide decision support to local authorities when it comes to developing new strategies for adaptation to climate change.
Climate informatics: an emerging discipline for the future
Claire Monteleoni has been something of a pioneer when it comes to combining machine learning and climate science. In 2008 she attended a seminar held at Columbia University by Gavin Schmidt, a leading climate scientist at NASA who was keen to spark debate about the huge volumes of data and the need to collaborate with computer science. Claire posed the following question during his talk: “Have you tried machine learning for these problems?” It was a good question to ask. After all, machine learning (which along with methods from optimisation and statistics provides the algorithmic foundations for much of modern artificial intelligence) had already proved its worth for forecasting, including in the stock market, combining predictions from multiple experts. It was also becoming increasingly commonplace in industry and medicine - so why not use these methods to combine the predictions from the climate models employed by the IPCC (Intergovernmental Panel on Climate Change)?
This was the idea posed by Claire and Gavin, and their results were subsequently discussed by the IPCC. At a NASA conference in California in 2010, their paper was awarded the best paper prize (Monteleoni et al., 2010). Then, in 2011, they founded of the International Conference on Climate Informatics which brought together an international network of scientists to form a new research community called climate informatics.
Machine learning is the key to unlocking insights in the massive amounts of existing climate data.
Claire believes that AI is the most cost-effective way of learning from the massive volumes of data which have already been collected, whether through digital simulations, or observed via remote sensing and in-situ measurements. Meanwhile, one advantage of machine learning is that the more data there is to analyse, the better AI’s performance becomes!
The ARCHES project: Leveraging AI for Climate Change and Environmental Sustainability
Claire’s research group showed that a variant of convolutional neural networks, a type of deep learning, could provide an effective way of forecasting the path of hurricanes, events which are very difficult to predict (Giffard-Roisin et al., 2020).
This work has already been highly cited, but there remain many open questions. For example, Hurricane Patricia, which struck the west coast of Mexico in October 2015, suddenly went from a category 1 hurricane to a category 5 “monster” (with wind speeds shifting from 138 km/h to 333 km/h in the space of 24 hours). Scientists are still at a loss to explain these sudden changes in intensity.
AI could help improve forecasting, both short-term and long-term. It could also be used to tackle other challenges society will face in the future, such as choosing where to live, which types of houses are best and the necessary public infrastructure, as well as assessing the reliability of solar and wind energy production. In short, AI could help us to better predict our climate future, ensuring that we are capable of adapting and being resilient when faced with change.
Claire Monteleoni plans to name her project team “ARCHES”, which stands for “AI Research for Climate CHange and Environmental Sustainability.” Research will focus on three main areas:
- AI for climate science: to improve scientific understanding of how the climate system is changing.
- AI for adapting to climate change: to consider the social impact and to assist communities and decision-makers by providing decision-support tools.
- AI for mitigating climate change: to accelerate our ecological transition, with a particular focus on renewable energy.
“There are many other problems for which machine learning could be used, and we encourage others to get involved”, explains Claire Monteleoni, who has helped students get interested in climate data science by staging hackathons:
It’s fantastic to see a whole generation of data scientists and AI researchers, particularly students, take an interest in climate informatics.
Through these areas of focus, the ARCHES project will represent a major breakthrough in the use of artificial intelligence to address issues related to climate change and environmental sustainability.
The research that will be carried out by the ARCHES project will aim to help scientists better understand the climate, facilitate adaptation and resilience to change, and accelerate the green transition. By encouraging interdisciplinary collaboration between climate science, renewable energy, machine learning, data mining and statistics, ARCHES hopes to pave the way for major advances in the fight against climate change.
About Claire Monteleoni
Claire Monteleoni is holder of the Choose France AI chair and director of research at the Inria Paris centre. She is professor in the Computer Science department at the University of Colorado Boulder.
In 2023, as part of the Choose France programme, Claire Monteleoni joined Inria to form a new research team which will operate in the field of machine learning in climate informatics.
Claire had previously occupied positions at Paris-Saclay University, at the CNRS (from 2017 to 2018 as part of the “Jean d’Alembert” fellowship programme), at the George Washington University (from 2011 to 2018, first as an assistant professor and then as an associate professor) and at Columbia University (from 2008 to 2011, as a research scientist).
She completed her Masters and her PhD in Computer Science at MIT in 2006 and was a postdoctoral researcher at UC San Diego between 2006 and 2008. She holds a Bachelors degree in earth and planetary sciences from Harvard.
Claire Monteleoni's research on the use of machine learning for studying climate change helped to launch the interdisciplinary field of climate informatics. She co-founded the International Conference on Climate Informatics, which held its 12th edition in 2023 and which has been attended by climate scientists and data scientists from more than 20 different countries and 30 U.S. states.
Claire currently serves on the advisory committee for environmental research and education, at the NSF (U.S. National Science Foundation) and is the founding editor-in-chief of the journal Environmental Data Science, which was launched in December 2020 by Cambridge University Press.
Bibliography
- Sinha, S., Hodge, B., & Monteleoni, C. (2022). “Week-ahead solar irradiance forecasting with deep sequence learning”. Environmental Data Science: Special Collection: Proceedings of the 12th International Conference on Climate Informatics 2022, 1, E28.
- Brian Groenke, Luke Madaus, and Claire Monteleoni (2021). “ClimAlign: Unsupervised statistical downscaling of climate variables via normalizing flows”. In Proceedings of the 10th International Conference on Climate Informatics (CI2020). Association for Computing Machinery, New York, NY, USA, 60–66.
- Saumya Sinha, Sophie Giffard-Roisin, Fatima Karbou, Michael Deschatres, Anna Karas, Nicolas Eckert, Cécile Coléou, and Claire Monteleoni (2021). "Variational Autoencoder Anomaly-Detection of Avalanche Deposits in Satellite SAR Imagery”. In Proceedings of the 10th International Conference on Climate Informatics (CI2020). Association for Computing Machinery, New York, NY, USA, 113–119.
- Giffard-Roisin Sophie, Yang Mo, Charpiat Guillaume, Kumler Bonfanti Christina, Kégl Balázs, Monteleoni Claire (2020). “Tropical Cyclone Track Forecasting Using Fused Deep Learning From Aligned Reanalysis Data”. Frontiers in Big Data. Volume 3.
- Monteleoni, C., Schmidt, G. A., Alexander, F., Niculescu-Mizil, A., Steinhauser, K., Tippett, M., et al. (2016). “Climate Informatics”, Comput. Intell. Data Anal. Sustain. Dev., 81–126.
Videos featuring Claire Monteleoni
- Lecture (Environmetrics Webinar, 2023): Claire Monteleoni: Machine Learning for Climate Change and Environmental Sustainability.
- Interview (Cambridge University Press, 2023): Meet Claire Monteleoni: Editor in Chief of Environmental Data Science.
- Interview (TWIML AI Podcast, 2021): Deep Unsupervised Learning for Climate Informatics with Claire Monteleoni.
- Interview (CU Boulder Engineering & Applied Science, 2020): CU Boulder Engineering Faculty: Claire Monteleoni.
- Filmed profile (University of Colorado Boulder, 2020): Machine Learning and Climate Change: Research and Innovation Office Community Talk.
- Lecture (NeurIPS 2014): Climate Change: Challenges for Machine Learning.