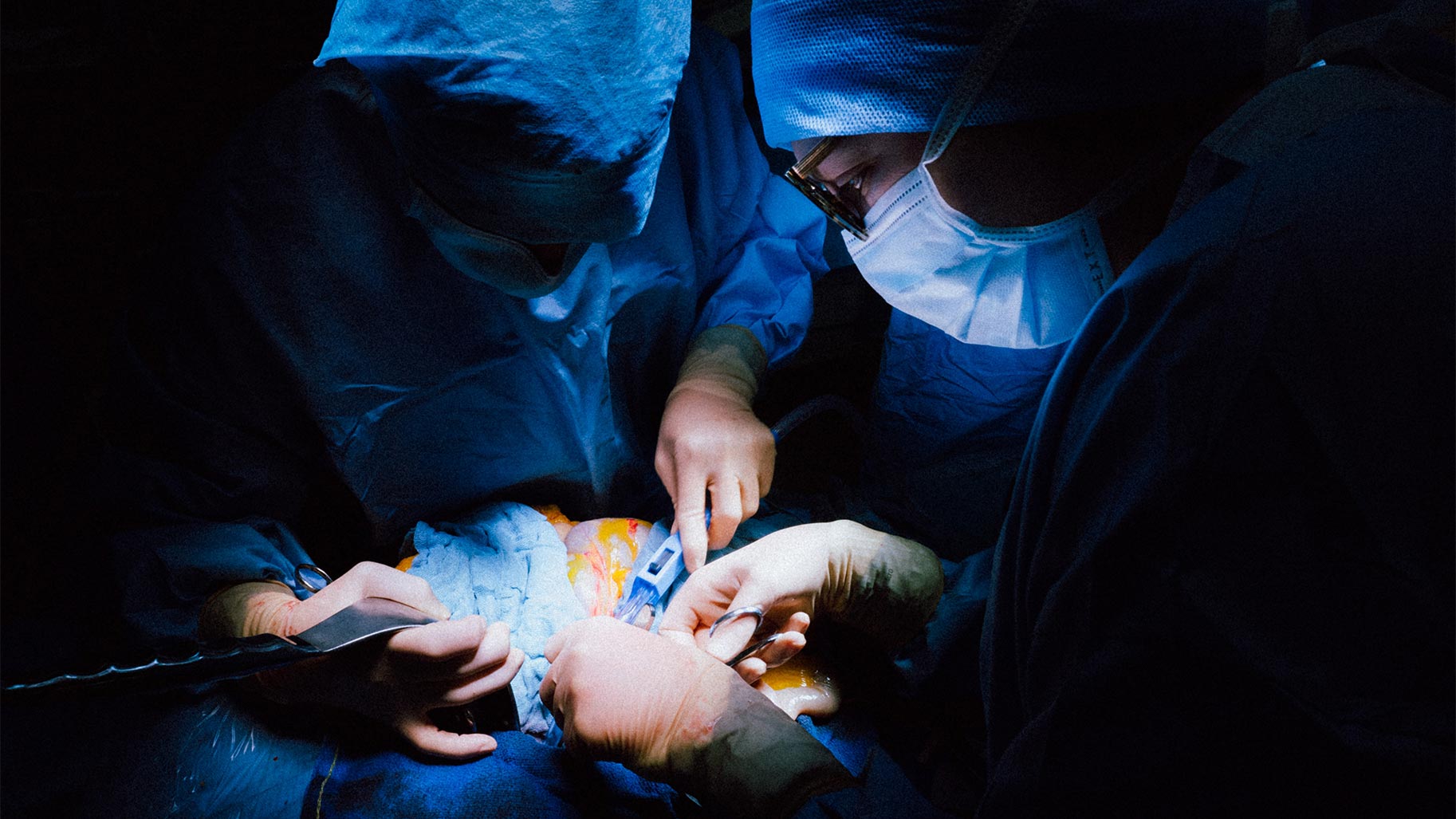
At the crossroads between computer science and healthcare
At the end of 2017, I had a visit from Professor François Pattou”, says Philippe Preux, Director of the ScooL project team at the Inria centre at the University of Lille. “As a surgeon at Lille University Hospital, Director of an Inserm unit and a professor at the University of Lille, he had been collecting data since 2006 on the results of his bariatric surgeries and wanted to know if it could be used in some way to improve patient care. The idea appealed to me because it was a great challenge!
Professor Pattou had approached the right person: the Scool project team specialises in sequential decision-making under uncertainty. In other words, it aims to model the consequences of a series of decisions as the environment in which they are made changes. Predicting the effects of surgery on a patient, an environment in constant evolution, was indeed within its ambit. “However, at the end of 2019, we were just two professors with the desire to do something on the subject… but we needed the means,” says Philippe Preux.
Fully personalised modelling
The two researchers responded to various calls for proposals and, in late 2018, were granted €110,000 by the I-Site ULNE (Université Lille Nord Europe) foundation. This was enough to pay a PhD student, Patrick Saux. Other funding, in particular from Inria and the European project Sophia, allowed them to strengthen the team by hiring an engineer for data analysis and a biostatistician.
All that remained was for the work to begin... which was not that simple! “We wanted to work together and we had the data, but in such a multidisciplinary context we needed time to get to know and understand each other. We also still needed to answer one specific question”, says Philippe Preux. “What could we measure? What could we predict that would be useful for doctors and their patients?” The process of reflection took over a year but eventually allowed them to identify a problem of interest: predicting the pertinence of post-operative visits, in the short and long term, for each patient.
“The key word behind this idea is personalisation”, says Philippe Preux. “It is about knowing whether, for a given individual, the cost of a visit in terms of mobilisation of doctors, patient travel, etc., can be optimised in relation to what it offers: detection of a possible complication”. In mathematics, this is known as the multi-armed bandit problem: how to optimise a reward in relation to a cost for a series of decisions with uncertain consequences. “But in our case there is a specificity, because the two options - to summon or not to summon the patient - are not symmetrical”, explains Patrick Saux. “Particular importance is given to the risk, and given the uncertainty, it is better to summon the patient”.
First stage: predicting weight loss
The key is in the project name: B4H, Bandits For Health. But before employing bandits for the optimisation of visits, the team must have tools to assess patients’ conditions and the risks of complications. The researchers laid the first foundations by creating algorithms capable of predicting, in a personalised manner, the expected weight loss after bariatric surgery. “
Although we had extensive data for each patient, the number of patients was limited to just a few thousand”, says Patrick Saux. We compensated for this relatively small sample by using the expertise of doctors and dieticians. That’s the advantage of interdisciplinary work!
The model they developed produces a curve that is specific to each individual and describes the expected change in weight up to five years after surgery. This offers multiple advantages, first of all for the patient, who can actually see their future weight loss. “Some have unrealistic expectations before bariatric surgery. A visual aid helps to keep them in line with reality and makes it easier to accept the operation”, says Patrick Saux. Secondly, there is an advantage for the doctor because a significant deviation from the expected trajectory could mean a complication and therefore the need to reoperate or change the postoperative treatment.
Robust machine learning
“The algorithms used for this model are based on decision trees, which are series of questions concerning the patient’s age and weight, whether they smoke or not, whether they have diabetes, the type of surgery envisaged, etc.”, explains the Ph.D. student. “They are very robust, their predictions are relatively precise and they are also easy to interpret. This allowed the doctors to verify that the algorithms took account of sufficient criteria, which also had to be relevant, to make their predictions”.
Health experts also contributed to refining the smoothing algorithms: as the model was only created from punctually collected data (before surgery and then after three months, a year, two years, etc.), they had to fill in the gaps between the collection dates. “Again, doctors know what the weight curve usually looks like, so they were able to guide us in the right direction”, explains Patrick Saux.
This collaboration between computer scientists and healthcare professionals allowed them to produce an accurate model. As proof, it was successfully tested in various hospitals in the Hauts-de-France region and even on eight international cohorts, including one with 6,000 patients in the Netherlands. This validation stage led the team to create an initial website to allow the partners to enter their patients’ data and see the results. The ergonomics of the site were then reworked, again in concertation with the doctors, so that it could be used by general practitioners (GPs).
Towards automated decision making
The team who is working on B4H project is continuing to refine their model to make it dynamic, i.e. able to evolve over time according to data measured on the patient at each follow-up visit. This will ultimately make it useful for the deployment of the bandits. “We will be able to use the skeleton of this model to create others capable of predicting other complications, such as diabetes or undernutrition”, says Patrick Saux. “These different models will be combined by bandit algorithms that will allow the suggestion of action/decision making on the need for a visit, whether to the University Hospital or the GP or not.”
This is the challenge of the second part of Patrick Saux’s Ph.D., which will finish in 2023. In addition, the ANR (French National Research Agency) has granted funding for four years for the continuation of B4H after 2023… the bandits will be able to keep on running!
Find out more (in French)
- Obésité : le suivi est crucial après une chirurgie bariatrique, Le Figaro, 28/6/2018.
- Chirurgie bariatrique : aider les personnes obèses à améliorer leur projet de vie, AP-HM, Hopitaux universitaires de Marseille, 2/12/2015.