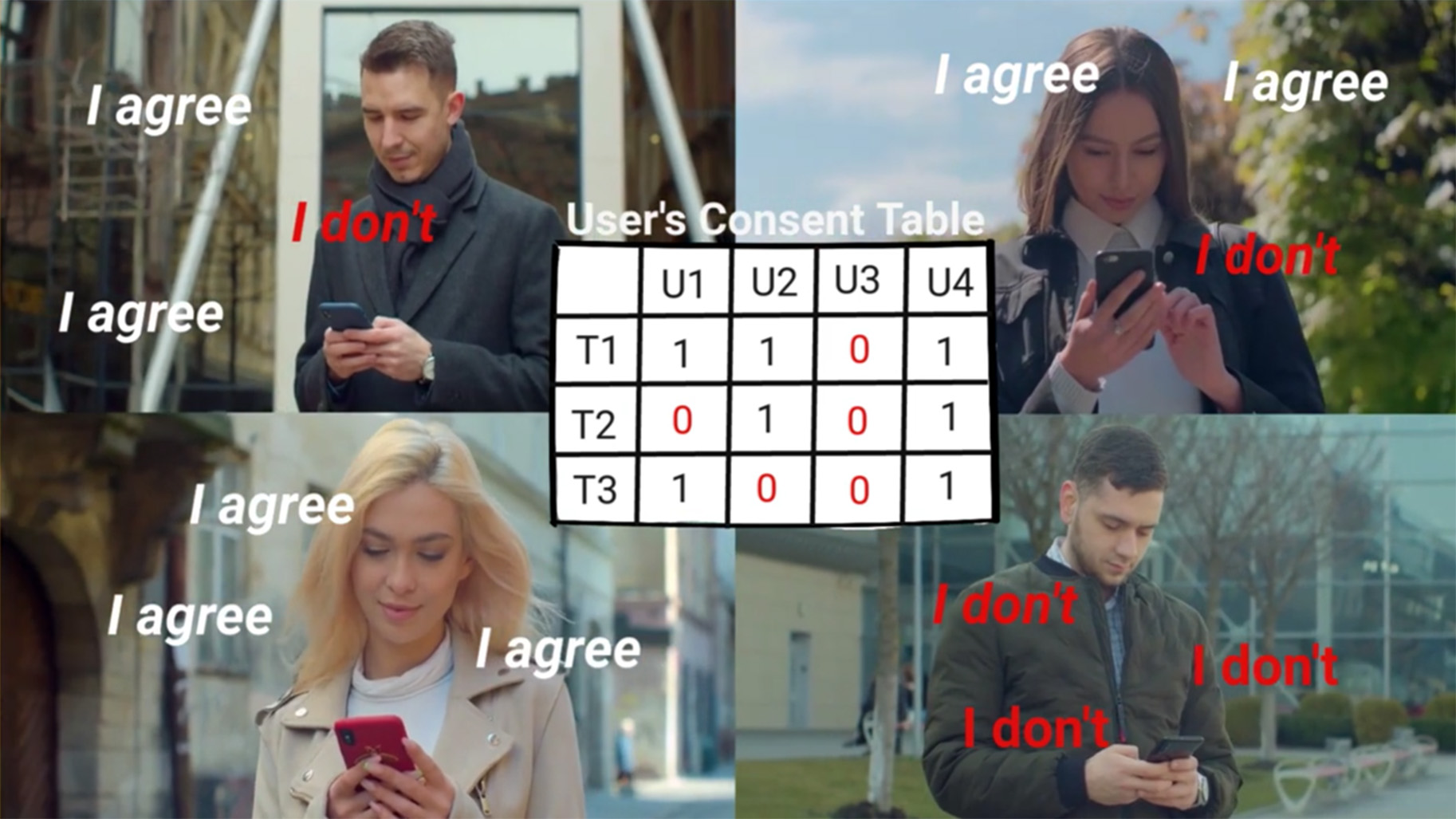
GPS antenna, microphone, camera, barometer, pedometer, gyroscope, QR code scanner, etc. Our modern-day telephones are embedded with a multitude of tools. On a planetary scale, this represents billions of sensors providing vast amounts of information on a wide array of subjects, such as road conditions, the bird count in gardens, underground vibrations or average hours of sleep for a given population.
Collected on a voluntary basis, this data is of great interest to all kinds of institutions and businesses, who use it to carry out wide-scale analysis inexpensively. Crowdsensing applications and platforms thus increasingly connect the participants supplying data with organisations using this information for environmental, societal, health or sales surveys, etc.
There is an issue lurking in the background; that of the reuse of data initially collected for a specific task, but which can potentially serve another one. Ideally, the procedure offers huge advantages. By reusing data which is already available, analysts save on the costs linked to collection. Moreover, by drawing on the mass of information accumulated over time, they have access to an enhanced data set for their analysis.
PETRUS project-team
The Inria Petrus project-team at the Université of Versailles Saint-Quentin-en-Yvelines, within the DAVID laboratory, works on the protection of privacy through software architecture design in future ‘personal clouds’, i.e. those spaces where individuals can store their holiday snaps, administrative documents or utility bills.
Personal data extracted despite the lack of consent
‘We examined the lack of consent when a user agrees to participate in certain tasks, but not others’, says Mariem Brahem, research engineer and co-author with Valérie Issarny (1) of a paper on the topic for the PerCom conference on pervasive computing. ‘We demonstrated that in certain cases a participant’s private data can be included without their consent in the data collected from a group.’
The study drew on a specific case involving 120 people who are asked to switch on their telephone microphone for a week in order to record sound pollution in a given area or when travelling through their city. Each user can give their consent either for a first or second task only, or for both.
Verbatim
We demonstrated that in certain cases a participant’s private data can be included without their consent in the data collected from a group
Research engineer in the PETRUS project team
And this is where things get surprising. Even if a user has only consented to a task which does not share their private data (e.g. their movements), it is still possible for the analyst to extract this personal data from that collected in a group, if this group consists of participants with more lenient consent.
In order to grow, crowdsensing thus requires the building of an additional layer of software to protect users from this risk. There are two aspects to the solution offered by Petrus researchers. ‘First, we designed a manifesto’, Mariem Brahem explains. ‘The user ticks boxes in this manifesto to clearly specify which task(s) they consent to, for which purpose and by whom. On this very detailed basis, the system must ensure this consent is complied with throughout all the data processing steps.’
Grouping participants according to their level of consent
Based on this, an algorithmic solution will measure the distance between the expressed levels of consent. It will then form groups of participants according to these levels. People with similar levels will find themselves in the same group. ‘If you consent to both task 1 and task 2, you’ll be in the same T1+T2 cluster.’ Each of these groups will also be of a minimum size. This will make it more difficult to extract information on a participant by comparing them to others. The algorithm is called l-complétude. ‘The l is the parameter which corresponds to the number of people in a group.’
To be wholly useful, however, this combination of tasks and consent must meet an additional requirement. Efforts must be made to produce groups which can execute a maximum of tasks. In other words, no participants should be left aside when their readings could be included for certain tasks in the data set under analysis. The algorithm will thus optimise this distribution between group creation and task attribution.
Via this proof-of-concept, the researchers hoped to demonstrate the benefit for crowdsensing applications to systematically incorporate this type of l-complétude property in their architecture.
Will there be a follow-up to these studies? ‘We’re still exploring lack of consent, but in another context; that of remote working.’ The widespread growth of this trend has escaped no-one, but it also raises new issues related to privacy. ‘Imagine, for example, that your employer agrees to reimburse your electricity costs linked to your professional activity. You therefore need to provide them with your meter readings. At the same time, you don’t want to disclose information on your electricity use for other household activities. Your employer doesn’t need to know if you put the washing machine on. We thus need to find a solution so that each person can define their level of privacy.’
----
(1) Valérie Issarny was an Inria researcher specialised in distributed systems. She died last November following a long illness.