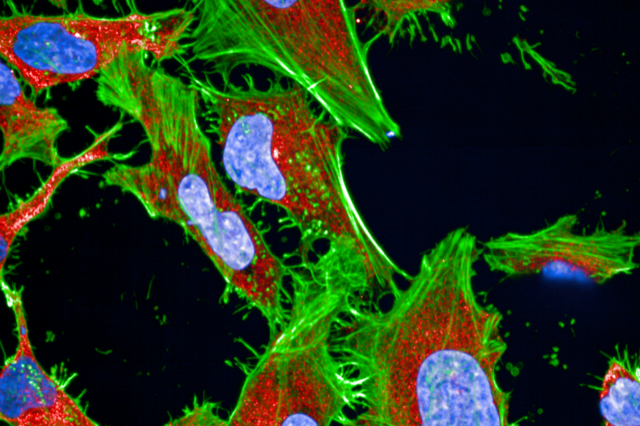
CIFRE theses: an academic and industrial partnership
“This CIFRE* thesis is actually the realisation of an exploratory systems biology project that we started at Inria in 2001,” laughs François Fages, director of the Inria Lifeware project team. “This proves that the project is bearing fruit!” In 2017, Jeremy Grignard picked up the baton on this topic, during a research internship with François Fages’ team as part of his IT and Big Data Engineering studies at the École Centrale d’Électronique. He then went on to complete a CIFRE thesis, combining two partners, academic and industrial. While Inria Saclay Centre was on board to fill the first role, Jeremy Grignard had to find the second and prospected various companies, including pharmaceutical industrials, pitching them the idea for his thesis: machine learning for models using experiments and temporal data.
Servier welcomed him, as within the idea of modelling lies a sizeable challenge for the pharmaceutical industry: to better understand biochemical processes and signalling pathways involving a dysregulated therapeutic target in an illness to better identify drug candidates that could modulate it. And therefore save time and money. Over 58% of drug candidates fail in phase III (trials of large cohorts of patients with the illness to be treated). This represents the loss of years and billions of euros of investment.
Mathematical modelling to understand biological processes
“A large part of what we needed was investigation, which lent itself well to the thesis,” explains Thierry Dorval, director of the Data Science and Data Management Department at Servier. “And there was no shortage of experimental data for the research!” The question was, how to give this data meaning and best exploit it.
Jeremy Grignard started with a concrete case: molecules developed by Servier have an effect at the biochemical level on a protein involved in a particular process (microtubule tyrosination), but this activity is not found at the cellular level. And the researchers did not understand why. The young engineer therefore developed an expert mathematical model using laboratory data, which provided the explanation: the biological process is based on two reactions, and activating only one is therefore not enough!
The model also revealed another protein, which, when inhibited, made it possible to achieve the desired result... opening up a new avenue for researchers. “The idea is now to develop this type of model for each therapeutic project,” says Thierry Dorval. “This involves studying machine learning for models... and it will be the subject of a future second thesis.”
A reoriented thesis, normalised data
While this theme was at the heart of Jeremy Grignard’s thesis from the beginning, the topic has evolved slightly. “We realised that temporal data, which was meant to be the foundation for Jeremy’s research, was not the kind of data that we had the most of,” continues the Servier specialist. “There was a lot more imaging data.” Jeremy Grignard’s thesis was therefore renamed “Computational Methods to Improve Early Drug Discovery” and the researcher focused on using imaging data. In particular, he worked on phenotypic screening experiments.
This involved staining cells, then using image analysis software to observe modifications in their appearance (phenotype) in response to a genetic disruption or chemical molecule. “The problem resides in the large number of variables measured, which we need to be able to normalise in the hope of then connecting a phenotypic modification with the activity of a molecule or genetic disruption,” emphasises Jeremy Grignard. And so, he developed two algorithms to perform this normalisation.
Their potential application is huge, as Servier is part of the JUMP-CP international consortium, which brings together ten major pharmaceutical companies under the aegis of MIT and Harvard’s Broad Institute. The aim of this organisation is to undertake an enormous phenotypic screening experiment by studying the effects of 120,000 chemical molecules and CRISPR/Cas9 genetic disruptions. Using the normalisation algorithms developed by Jeremy Grignard, and creating others, will make it possible to exploit the big data generated.
Linking and relinking data to better exploit it
But the question remained: how? The answer: through the knowledge graph. This mathematical tool makes it possible to integrate very heterogeneous data in very large volumes, as well as the relations that connect them, in a software capable of processing it all. The software developed by Jeremy Grignard is called Pegasus: today, it contains approximately 46 million nodes, i.e. data, from internal Servier research or the public domain, as well as 331 million relations between this data.
While such numbers make the tool seem abstract, its applications are certainly concrete. For example, Pegasus has already been used to select antisense oligonucleotides (ASOs), small genetic sequences capable of altering the expression of a target protein in a rare illness: epileptic encephalopathy. The future objective is to enable Servier researchers to quickly identify potential ASOs for a given therapeutic target.
Pegasus also makes it possible to identify molecules developed by Servier that are chemically similar to molecules studied in scientific publications for their effect on a therapeutic target. “Instead of screening a large number of molecules to see if they act on the target, which on average leads to a 1% success rate, we could base ourselves on similarity connections found in Pegasus to screen the most interesting molecules directly. This would significantly increase the success rate and quality of molecules identified,” states Jeremy Grignard.
Three complementary, scalable tools
The three tools are perfectly complementary: Pegasus is based on data from mathematical modelling and phenotypic screening experiments that the algorithms can normalise. We have come full circle... or almost. Because the databases are huge, and Pegasus, as well as the related industrial applications, are likely to evolve.
This is also why Jeremy Grignard was hired immediately after the three years of his thesis, in March 2022, as a data scientist at the Servier research institute. ‘CIFRE theses make it possible to answer very concrete questions posed by an industrial company, by developing theoretical approaches that we would not have thought of ourselves,” says François Fages. “Furthermore, the tools developed can open up new avenues for us: we have several research projects for which Pegasus could be very useful.” The collaboration sparked by the CIFRE thesis is certainly not over...
* Industrial agreements for training through research
Find out more
- La Méthode scientifique avec Jeremy Grignard, France Culture, 12/1/2022 (in French).
- Servier & Pegasus - Graphe de connaissances pour supporter la recherche de nouveaux médicaments, Neo4j, 8/4/2022 (in French).