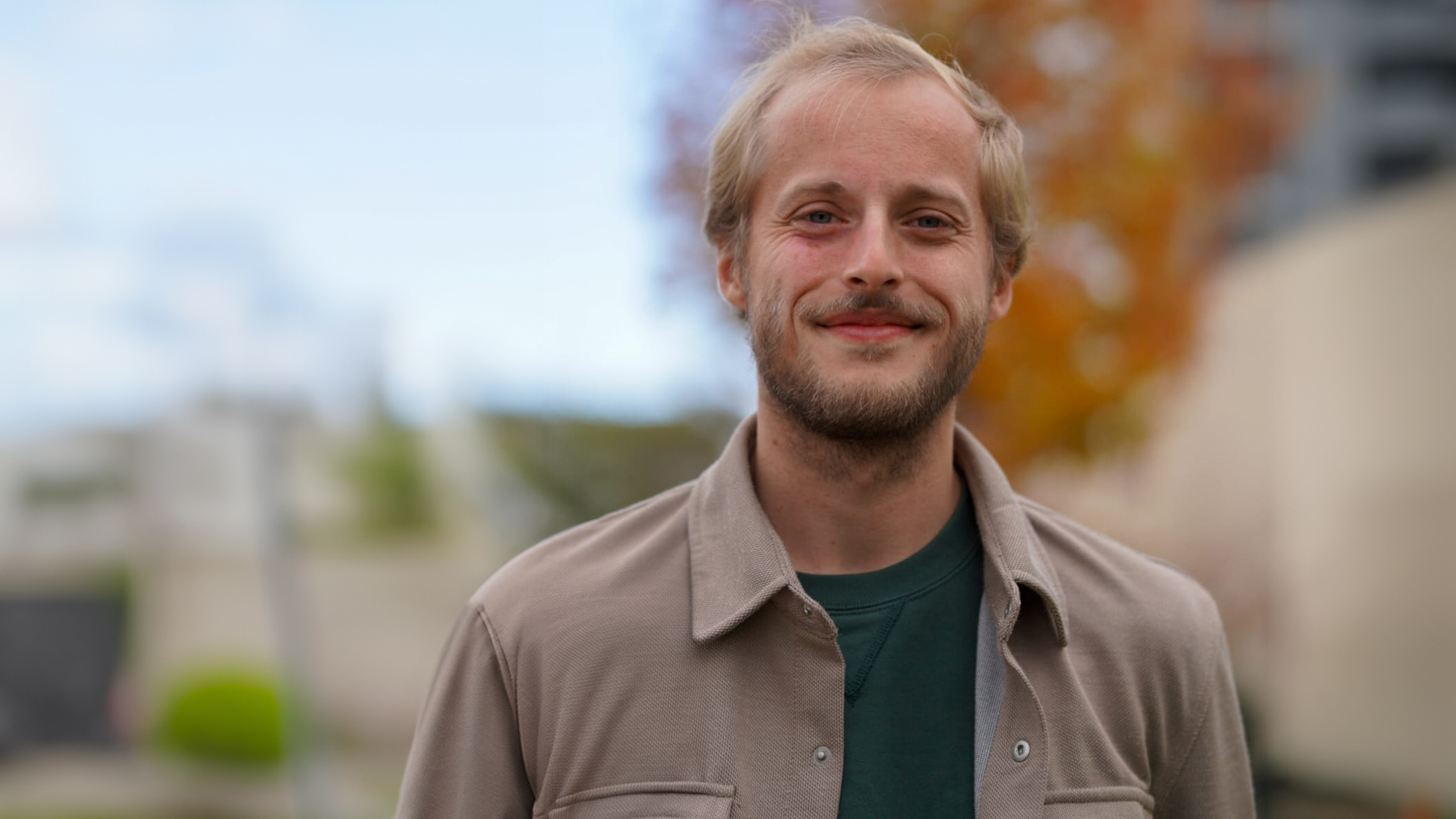
A gradual progression
Having grown up in Toulouse, the birthplace of Airbus and home to the CNES (French National Centre for Space Studies), Paul Boniol could have opted for a career in aeronautical engineering, a sector which he was drawn to in his younger years. But after studying for his entrance exams for France’s Grandes Ecoles, he ended up going down a different path: “I was accepted by the ENSIMAG in Grenoble [a prestigious "Grande École" specialising in computer science and applied mathematics], where I first encountered computer science, and theoretical computer science in particular”, says the 29-year-old researcher.
Paul’s career progressed gradually from there, as he built relationships, worked on research projects and carried out field experiments. It was during his Erasmus year in 2016-2017 at the School of Computer Science and Statistics at Trinity College Dublin that he developed an interest in scientific research. Another key moment came when Paul met his director of research Themis Palpanas during a research internship with the LIPADE laboratory at Paris Cité University in 2017. They worked together to come up with the subject for Paul’s PhD: anomaly detection through time series analysis.
What is a time series?
A time series is a value that changes over time, for example a temperature curve measured over several years. How can we identify early, accurately and quickly the symptoms that could anticipate future anomalies in a system before they occur? How can we make such a meaningful prediction on the scale of a second? Paul's work focuses on this problem: automating the analysis of these large volumes of data by developing algorithms to better anticipate - and better understand - when a power station, wind turbine or dam is likely to break down, so that we can intervene earlier. (Image taken from the video « Des algorithmes prédictifs » - Université Paris Cité).
Anomaly detection: from theoretical research to practical applications
Paul Boniol spent three years (2019-2021) working on new unsupervised learning methods for creating algorithms capable of quickly detecting anomalies in large datasets. When most people think of data, they think of social media, the internet and privacy, but this theoretical research opened up a whole host of other applications. This attracted interest from EDF, with whom Paul studied for his PhD as part of the Cifre scheme, a French initiative enabling students to study for PhDs within companies.
Paul Boniol found the experience of doing a PhD funded by a company hugely rewarding: “EDF works with the best part of 1,500 people in R&D, including 160 PhD students. It wasn’t so different from working within a traditional research lab, and I was able to get to know people involved in the practical side of things.” One example of the work Paul did while studying for his PhD involved using data from sensors at power plants (e.g. hydraulic dams, wind farms or nuclear power plants) to identify anomalies with a view to optimising the maintenance of these facilities. This led to two patents being filed, one at a national level and another at a European level.
Although he remains a theoretical researcher, Paul also enjoys exploring the practical side of things. His postdoctoral fellowship at the IPGP (the Paris Institute of Planetary Physics) focused on volcanic eruptions, and he has also spent time as a guest researcher at the European Gravitational Observatory.
A new recruit for VALDA
“Ideas don't come to me when I’m sitting on the couch. I need to be working with other people.” It was in the hope of continuing this type of constructive dialogue that Paul Boniol joined VALDA, a joint undertaking involving Inria, ENS-PSL and the CNRS. His job is to provide temporal analysis for the research carried out by the team on the extraction of large quantities of data. Becoming a permanent researcher with Inria will give the young researcher the time and the resources to really focus on his work, within an institution “with an impressive reputation in the world of research”.
“Who dares wins!”
We asked Paul if his PhD prizes played any part in him joining Inria. “They certainly gave me a level of visibility, as well as opportunities to present my research and to meet other researchers.” The researcher also knows how important it is to avoid being too harsh on yourself, as you can often be your own worst critic. “I’m still working on my self-confidence. But it’s a case of who dares wins. You need to put yourself out there, come up with papers, and trust people who think you could win prizes.” And who knows? Maybe one day it will be a Paul Boniol book on the bedside tables of young computer science researchers, just like his own well-thumbed copy of Nonlinear Time Series Analysis by Kantz and Schreiber.
VALDA: extracting value from data
A joint undertaking involving Inria, the École Normale Supérieure de Paris (ENS-PSL) and the CNRS, the VALDA project team is made up of around 15 members. They explore systems for managing complex data and the fundamental aspects of this process, with an emphasis on data produced through human activity. The end goal of their research is to optimise complex resources such as time and space for processing so as to be able to extract value from them. The main fields of application relate to personal information management systems (PIMS), which help to give users greater control over their personal data: emails, calendars, contacts, search histories, travel information, etc.
Find out more about Paul Boniol and his research:
Find out more about his research:
- Paul Boniol's page.
- Thesis: "Detection of anomalies and identification of their precursors in large data series collections" (2021).
- Video: "Paul Boniol, multi award-winning post-doctoral researcher for the creation of predictive algorithms" (2023 - Université Paris Cité).