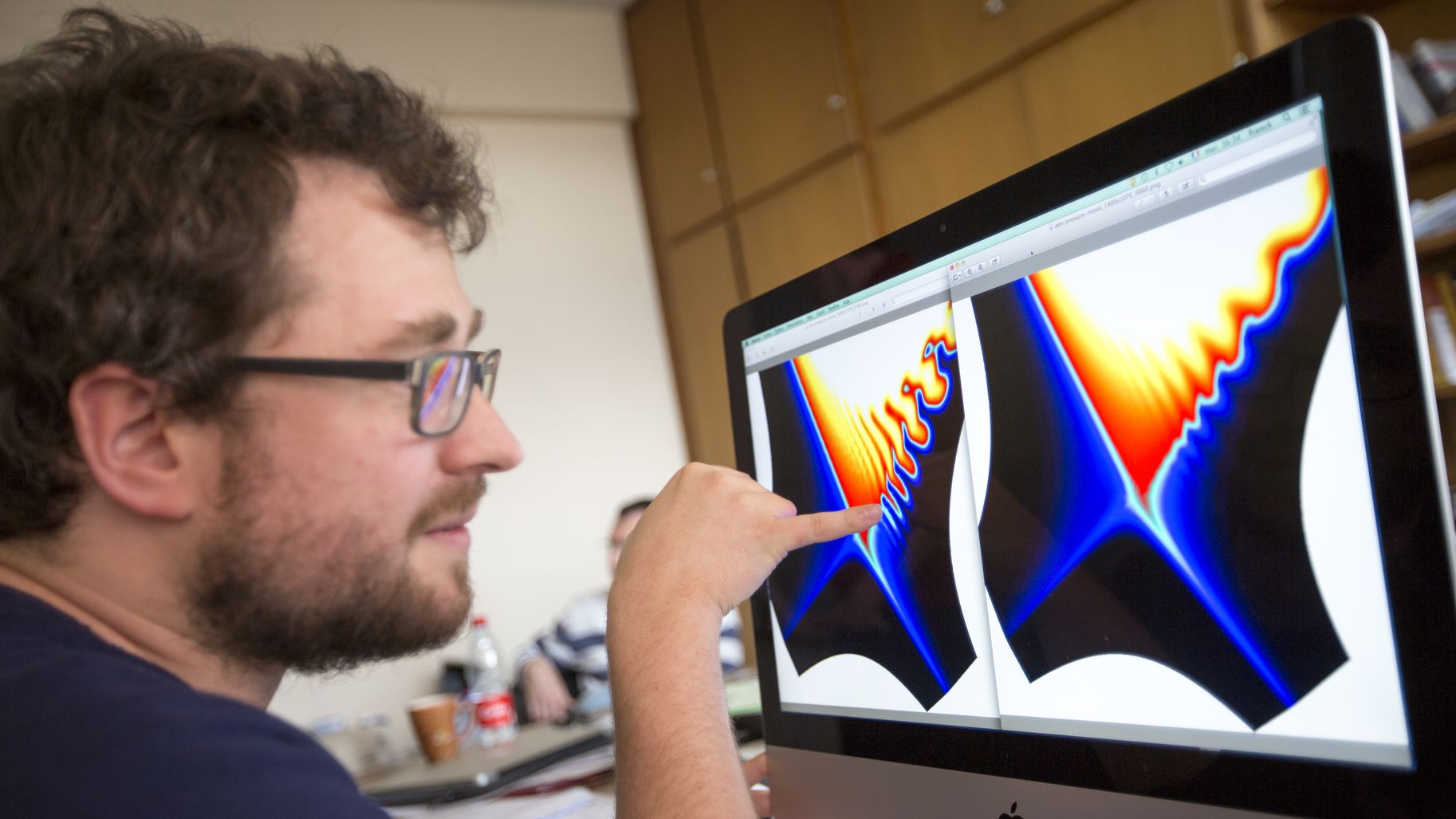
Nuclear fusion: how to simplify plasma simulation
Using innovative computer tools to model a source of energy that doesn’t exist yet - this is how we could describe Malesi (MAchine LEarning for SImulation), an exploratory action launched at the start of 2020 for four years and headed by two teams: Tonus and IRMA, the CNRS mathematics laboratory, both in Strasbourg.
Artificial intelligence: from consumer applications to hard science
“Exploratory” is just the right word to describe the level of risk for this project because it is still not certain whether nuclear fusion will meet expectations: providing an almost inexhaustible source of energy that releases little toxic waste. Similarly, it wasn’t until 2018 that the scientific community started to explore the use of artificial intelligence (AI) tools to render these models more reliable. Everything is yet to be invented!
“GAFA have been developing AI for several years, mainly for consumer applications”, underlines Philippe Helluy, Head of the Tonus team. “We’re now going to use it in hard science: it’s an exciting prospect which may profoundly change our work".
Five to 15 times more energy produced than consumed
Nuclear fusion, or the fusion of atomic nuclei, is a physical reaction that takes place in the heart of stars and which releases a huge amount of light and heat. To reproduce the process on Earth, deuterium and tritium atoms have to be heated to 100 million degrees and submitted to a pressure of 10,000 bars in ring-shaped fields in devices called tokamaks.
The atoms form a "soup" of matter (neither solid, liquid or gaseous), called plasma, that would destroy the walls of the enclosure at the slightest contact. Strong magnetic fields must therefore be used to keep it in the middle of the ring. The modelling process aims to predict the plasma’s behaviour as a function of temperature, pressure, magnetic fields and the tokamak’s geometry. The stakes are high. If "terrestrial" nuclear fusion works, it will generate 5 to 15 times more energy than it consumes!
Simpler models lack robustness
In recent years, physicists have developed reliable and accurate plasma models. But their size (they can have as many as 1,000 billion mesh points) makes them difficult to execute. “The CEA’s Gysela computing platform, which uses one million processors, can run for up to five weeks for just one simulation,” explains Emmanuel Franck, Head of Malesi.
Attempts have been made to simplify the models and reduce the calculations. But these less precise mesh methods generate less accurate physical results when they describe fast-evolving phenomena. The turbulence flowing through plasma can be transformed in a thousandth of a second and the temperature of the plasma can vary between 100 million degrees in the centre and 1,000 degrees at the edges. It’s hard to imagine less favourable conditions.
Using neural networks to make simpler models more reliable
The Malesi project has embarked on an audacious path to use AI to make these less complex models more robust. “Some teams have started exploring this avenue, for example by correcting the models used locally”, explains Laurent Navoret, Research Lecturer at IRMA. “Our aim is to improve our codes as a whole”.
In practice, researchers will use "convolutional" neural networks, used in AI for image analysis. These networks will scrutinize simulation results in the form of images, in this case clouds, each representing a value of physical parameters to be examined. “Initially, we will use clouds with reliable simulation points for what is called supervised learning”, says Emmanuel Franck. “Later on, the networks will analyse simulations carried out with simplified models which are more likely to contain errors”.
Is AI more powerful than numerical methods?
AI will play a dual role: first of all in detecting errors using what it learned in the previous phase, then, through successive repetitions, in correcting the parameters of simplified models until accurate results are obtained.
In addition, compared to numerical methods, the neural networks will be faster and more precise in performing interpolations, i.e. rebuilding the simplified images from point clouds. This is important because the execution of plasma models involves a lot of interpolation. “We have carried out very promising preliminary tests”, says Emmanuel Franck. “If they are confirmed, AI could correct the models while executing some of their tasks better”.
Nonetheless, prudence remains necessary at this stage. Malesi will have to verify that the AI tools do not damage the results obtained using standard methods. The other question is, will the use of neural networks actually save computing time, which was the project’s initial objective? “The response will largely depend on the learning phase”, answers Emmanuel Franck. “It is currently impossible to tell how many point clouds our neural networks will have to ‘learn’ in order to become operational.”