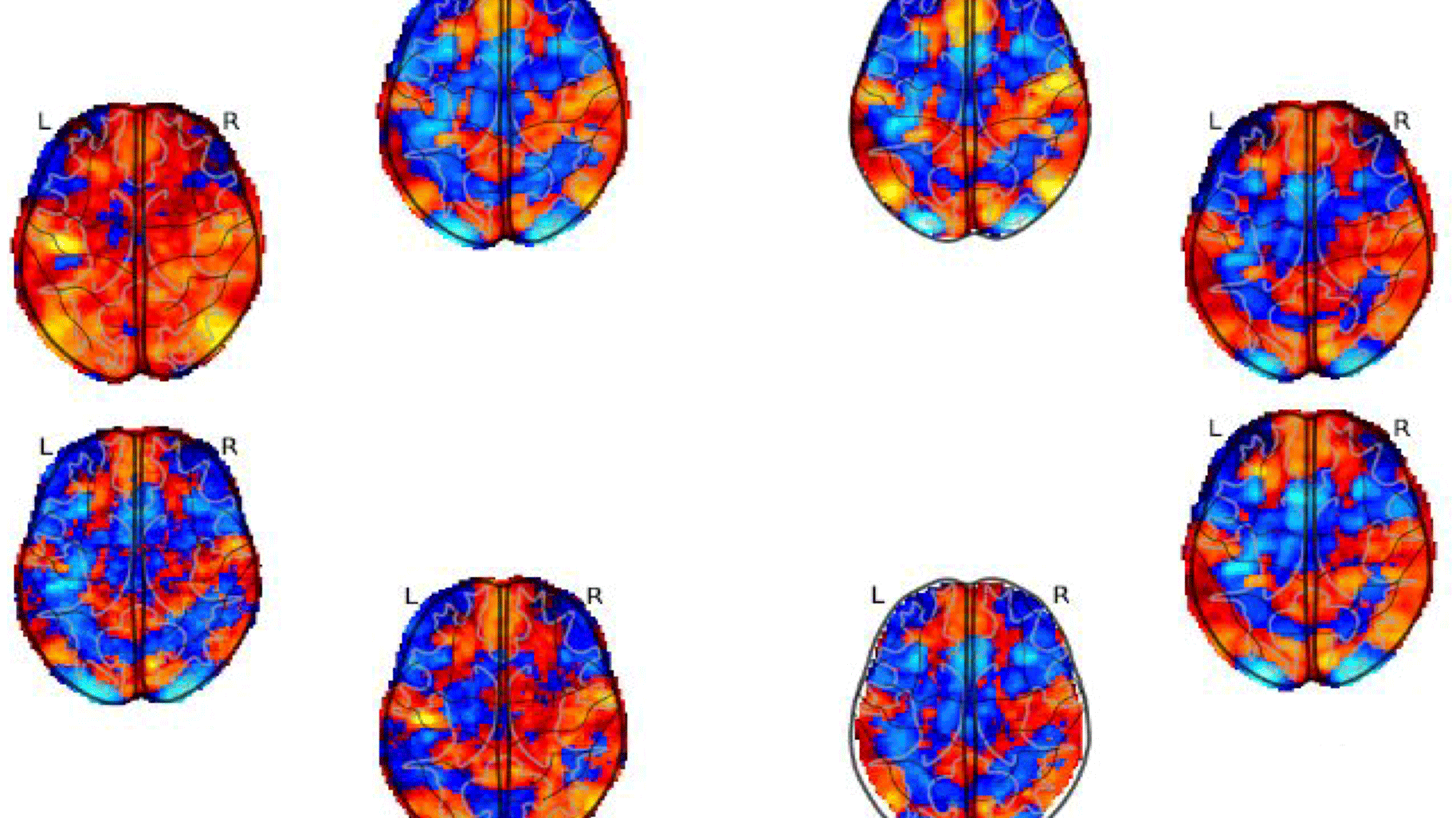
8 statistic maps of the same functional Magnetic Resonance Imaging (fMRI) experiment testing the negative parametric effect of loss for the equal indifference group in the gambling task of the NARPS project.
“A Waste of 1,000 Research Papers”. This was the somewhat provocative title of an article published in The Atlantic in 2019 on the topic of a study from the late 20th century that established the influence of the SCLA4 gene on depression. The article discussed the impact this major discovery had had on researchers in the field, and how the results had supported a good thousand papers over a period of 20 years. Then came the twist: as it turned out, the initial conclusions were false. And all of the research that had been built on top of them collapsed like a pack of cards. What this story teaches us is that the reproducibility of scientific results is a research topic in its own right. And neuroimaging is no exception to this issue.
In 2020 the scientist Rotem Botvinik-Nezer and several colleagues carried out an experiment for the journal Nature which involved giving the same dataset to 70 different neuroimaging pipelines, before carefully analysing the results that were produced. The aim was to determine whether or not carrying out a certain task was linked to the activation of an area of the brain. But not all of the pipelines came to the same conclusions.
This discovery was what prompted Camille Maumet to undertake an exploratory action aimed at reducing variability in processing chains. A member of the neuroimaging project team Empenn, Maumet's research concerns the reproducibility of results more broadly.
The key issue of data-sharing
The first question they had to deal with related to the data itself and the size of samples. “If you're analysing a relatively small group of participants then there's a greater risk of false positives. Another problem occurs when a sample isn't sufficiently representative of the general population. How many participants do you need in a study in order for it to properly represent human diversity?” To make matters worse, when it comes to rare diseases, databases put together locally will only contain a handful of cases. All of this speaks to a need for data-sharing in order to arrive at “samples which are bigger, more diverse and more representative of the population”. As part of a PhD jointly supervised by Camille Maumet and Elisa Fromont from the Lacodam project team (Inria/Université de Rennes/INSA Rennes/Institut Agro Rennes-Angers - Irisa), Elodie Germani awas able to put this approach into practice, demonstrating that models created using large, diverse databases have greater adaptability when it comes to new data.
Sharing is one of the cornerstones of the open science movement which many researchers ascribe to. But in order for data to be shared, it first needs to be shareable. And that’s where things get complicated. “A research team can publish their results online and say: help yourselves! But in practice, that’s not enough.” In fact, there are all sorts of questions which need to be addressed. “Can the format of the data be understood by other users? Are there enough descriptions to tell you how many participants the sample contains? How can you know that multiple images belong to the same person? If a specific protocol has been developed, is there a description of it? And so on.”
The conclusion?
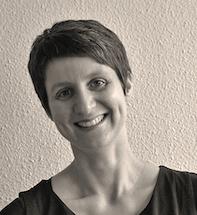
Verbatim
On its own data is often unusable: you need annotations, but that takes time. And scientists have enough on their plate as it is. You also have to annotate with a view towards making data reusable, but the space for annotation is huge, and so there are decisions to be made. Which specific annotations could be of use to others? I've done work on coming up with recommendations for this.
Research associate - EMPENN project team
Developing a code of conduct
There are also legal considerations to factor in, particularly within the EU where the General Data Protection Regulation (GDPR) outlines strict rules surrounding access to personal data. With this in mind, Empenn decided to join forces with other research teams as part of a project called OpenBrainConsent. “We developed multilingual document templates (in French in collaboration with Elise Bannier and Anne Hespel from Rennes University Hospital) which users must sign before starting a study. In accordance with this code of conduct, scientists agree to keep data anonymised, not to use data about people who have asked for it not to be used, and so on. There are still some practical questions that we will need legal experts to help us with in order to make sure that we can respect privacy while enabling science to progress.”
As part of OpenBrainConsent and alongside Cyril Pernet, from the University of Edinburgh, Camille Maumet launched an EU Cost action called GLIMR. The aim of this action is to pool data on the study of gliomas, a rare type of brain tumour for which samples within individual laboratories are extremely small. As a result, sharing is a necessity.
The second main issue is how robust the tools used are, and “how different ways of processing data affect the final result.” In reality, there are many factors which enter into the equation. “There are multiple stages of processing across a pipeline, with all sorts of choices to be made for each one, such as selecting the most appropriate tool. That tool might then have several algorithms, and each of those algorithms will have a series of parameters requiring further choices to be made. All of these choices have an impact on results. Obviously, experienced neuroscientists are able to whittle those choices down, but only to a certain extent.”
With the support of engineer Boris Clénet and postdoctoral researcher Jérémy Lefort-Besnard, the exploratory action Grasp will focus on this second aspect, drawing on the study carried out by Botvinik-Nezer. “It’s a really fascinating use case as you have real pipelines designed by researchers and a detailed description of the processing. We would also like to get the code for those 70 pipelines. By comparing the code with the description of the processing carried out and the result, our aim is to understand why a change in pipeline leads to a different result. Maybe the tool isn't working properly and no one has realised it. Maybe the selected pipeline isn't best-suited to addressing the problem. Or perhaps it wasn't configured correctly.”
Configuration can also cause phenomena of interest in datasets to either appear or disappear.
If I decide to add the variable linked to age and I get a different result, then that means that age is a factor which has an impact on the phenomenon I’m observing. In which case it’s the variability that has highlighted the problem.
By comparing the mechanisms at work in pipelines, it is hoped that the exploratory action will then be able to guide scientists in the choices they go on to make.
This is our second main area of focus. Our aim is to develop a statistical method that will inform researchers in neuroscience, warning them about analytical variability. Instead of launching one single processing chain, they can launch several. The idea is that our method will be able to produce a sort of overview: if you've got 10 processing chains, can you combine the results so you're left with just one ?
By utilising this vast array of tools, codes and configurations, it may even be possible to produce a logical model containing all of the possibilities available. “That isn't part of the exploratory action, but maybe that will be the next stage...”
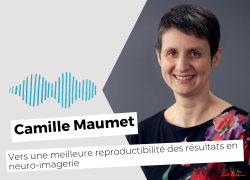
Know more about GRASP project (french)