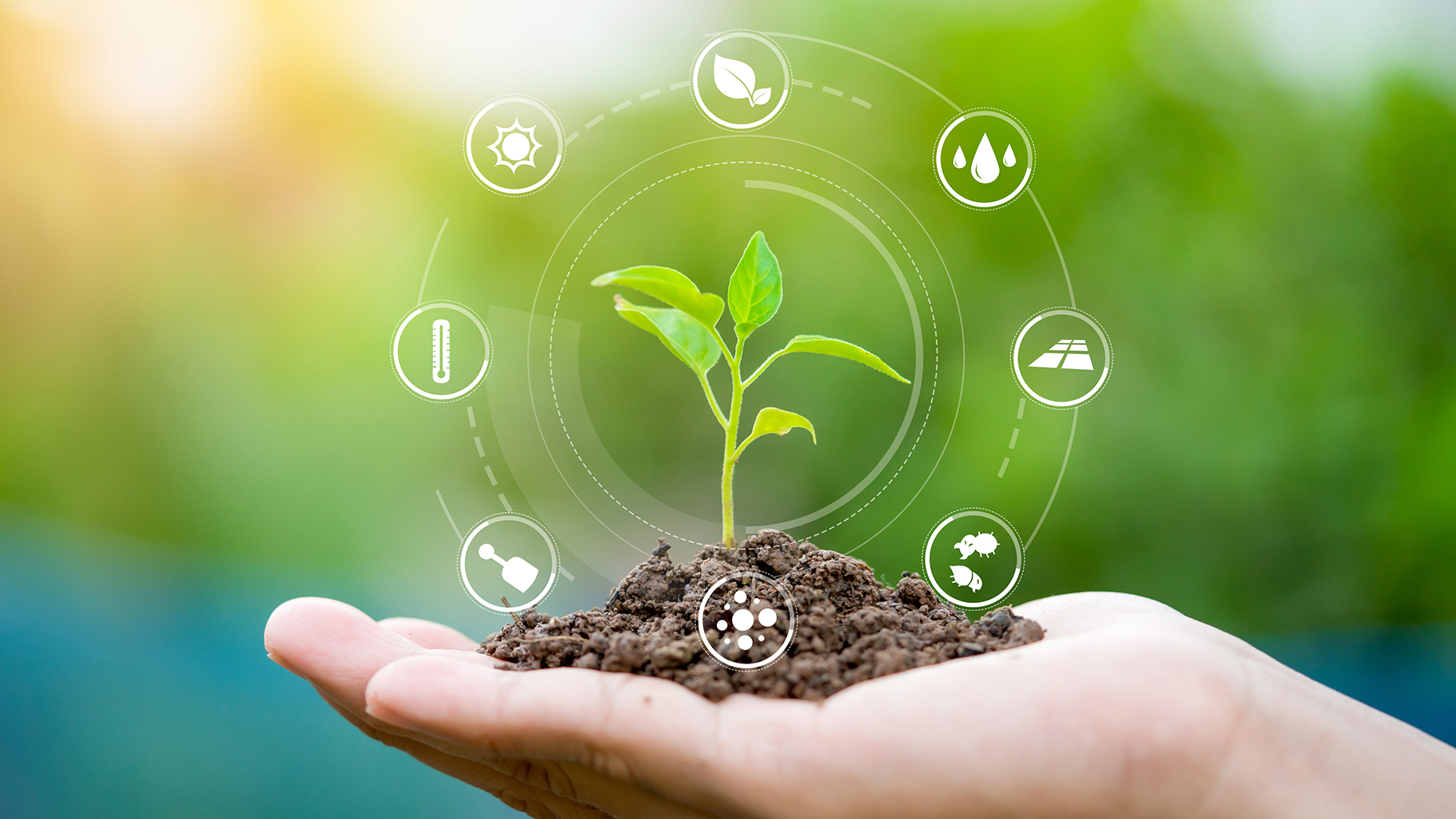
Laying the groundwork for a new subject
Since 2019, as part of Inria’s Exploratory Actions scheme, Odalric-Ambrym Maillard, a researcher from the project team Scool at the Inria center at the University of Lille, has spent part of his time working on a subject close to his heart - the agroecological transition - through the Inria Exploratory Action “Sequential Recommendation for Sustainable Gardening” (SR4SG). “When it comes to the use of digital technology in agriculture, people tend to think of robots replacing human expertise, with expensive tools requiring maintenance and fossil fuels, and which are limited to human perception. What I want to do is the exact opposite: low cost digital technology, employing intelligence in situations where humans lack the requisite data processing capacities”, explains the researcher.
Fundamentally, Odalric-Ambrym Maillard's research concerns machine learning, a form of trial-and-error learning similar to that used in experimental science. These types of algorithms, which were made famous by the victory of Google’s AlphaGo programme over a human player, have started to become a part of our lives since manufacturers began tackling these issues. It is these algorithms, for instance, which control the targeted content we see on social media, or which are used in driverless vehicles.
“The way I see it, my responsibility as an academic researcher is to consider future applications”, continues the researcher. Driven by personal motivations, Maillard’s vision for the future is centred around collaborative work, and it is here that the exploratory aspect comes into play. What the researcher has in mind is a crowdsourced tool which collects agricultural data in order to provide users with personalised recommendations (see inset) - a significant departure from the work currently being carried out in agricultural experimentation.
Building a network of agricultural partners
Agronomy is not in Maillard’s wheelhouse, however, and so he was forced to look beyond his own professional network. Seeking to apply his expertise in a field so different from his own, Odalric-Ambrym Maillard established ties with a number of agronomic institutions, including the French Agricultural Research Centre for International Development (CIRAD), which could be the first to test the new tool. His project also attracted interest from researchers in La Réunion, a region with a wide range of climates - and, therefore, a wide range of data from different climate zones - over a small surface area.
The idea was then to get farmers to volunteer to set up experiments. Rather than targeting certified organic producers, who have strict specifications they must adhere to, the researcher chose instead to focus on the experimenters from the Maraîchage sur Sol Vivant network, a community that has grown rapidly in France over the past ten years or so. Aside from the exploratory initiative, the researcher also attracted interest from a team of researchers at Bihar University in India, who were keen to try out an application for the daily collection of agricultural data, the aim being to develop a better understanding of agricultural practices and how they emerge.
As exciting as they were, these collaborations raised a number of questions: how can dialogue be enabled between the different communities using the tool? Which terminology should be used for plants, in a way that meets the varied needs and objectives of stakeholders? What’s more, conventional algorithms, like the one used to win at Go, operate in highly deterministic contexts, in which all parameters are known and visible. In agriculture, the context is anything but deterministic - you have no control over the weather for a start, then there is the quantity of observable parameters, and so on. As a result, compromises have to be made.
Another factor is that, unlike games, agricultural systems are autonomous. Regardless of what you do, plants continue to grow and insects and other animals continue to come and go. This raises the question: when is the best time to intervene? There are also questions linked to the learning framework for the algorithm, and exhaustive modelling isn't feasible as a result of the complexity of the system. As you can see, therefore, there are uncertainties with this situation. What could be done in order to develop a robust solution?
Seeking an answer to these questions, in addition to the work carried out by engineer Hernan David Carvajal Bastidas aimed at creating a prototype application, the exploratory initiative SR4SG has also funded a postdoctoral researcher, Timothée Mathieu, who was tasked with developing algorithmic and methodological solutions.
A highly promising tool
The challenges may be sizeable, but Odalric-Ambrym Maillard remains convinced: “If I put 20 years of my life into this project, it will be worth it, because the potential is enormous. But now is the time to act.” Maillard has been encouraged by the success of the application Plantix. This tool for recognising diseases and suggesting treatments is used by more than 10 million active users in India, a country where access to networks is more complicated than in Europe.
The exploratory initiative SR4SG will come to an end in 2023, but as far as the researcher is concerned, “it's already been a success. Software development is underway, and the prototype we have is sufficiently advanced for us to be able to demonstrate what we’re hoping to achieve.” And Odalric-Ambrym Maillard is already in discussions with Inria’s software development platform. Although the true impact of the project won't be felt for another few years, it will certainly be fascinating to keep an eye on its progress.
Moving forward together by sharing best practice
In agriculture, there are three types of people who carry out experiments. First, you have researchers working in the laboratories of experimental farms. Operating in a highly controlled context, they publish their results and employ a top-down approach to supply farmers with solutions. Whether or not these solutions are repeatable, however, will depend on individual soil contexts. Then there are the farmers themselves, whose scope for experimentation is limited by their need for productivity. They sometimes discuss their experiments with colleagues in their network. Lastly, there are amateur gardeners. Their experiments follow protocols which are often less rigorous, and tend not to be widely shared.
The goal for SR4SG is to build a collaborative tool for collecting data from these different practices (such as the techniques used and yields) and on-site observations (when pests arrive, when plants come into bloom, and so on). Once this data has been collated, the tool should be able to deliver personalised recommendations for practical use, based on similar contexts. These recommendations will then need to be adapted to the objectives of individual experimenters, whether it’s biodiversity preservation or short-term/long-term yields.
Find out more
- Agriculture: can digital help with the agroecological transition? Labo Société Numérique (the Société Numérique programme from the French National Agency for Territorial Cohesion), 25/3/2022.
- Agriculture and digital, Inria and Inrae white paper, January 2022.