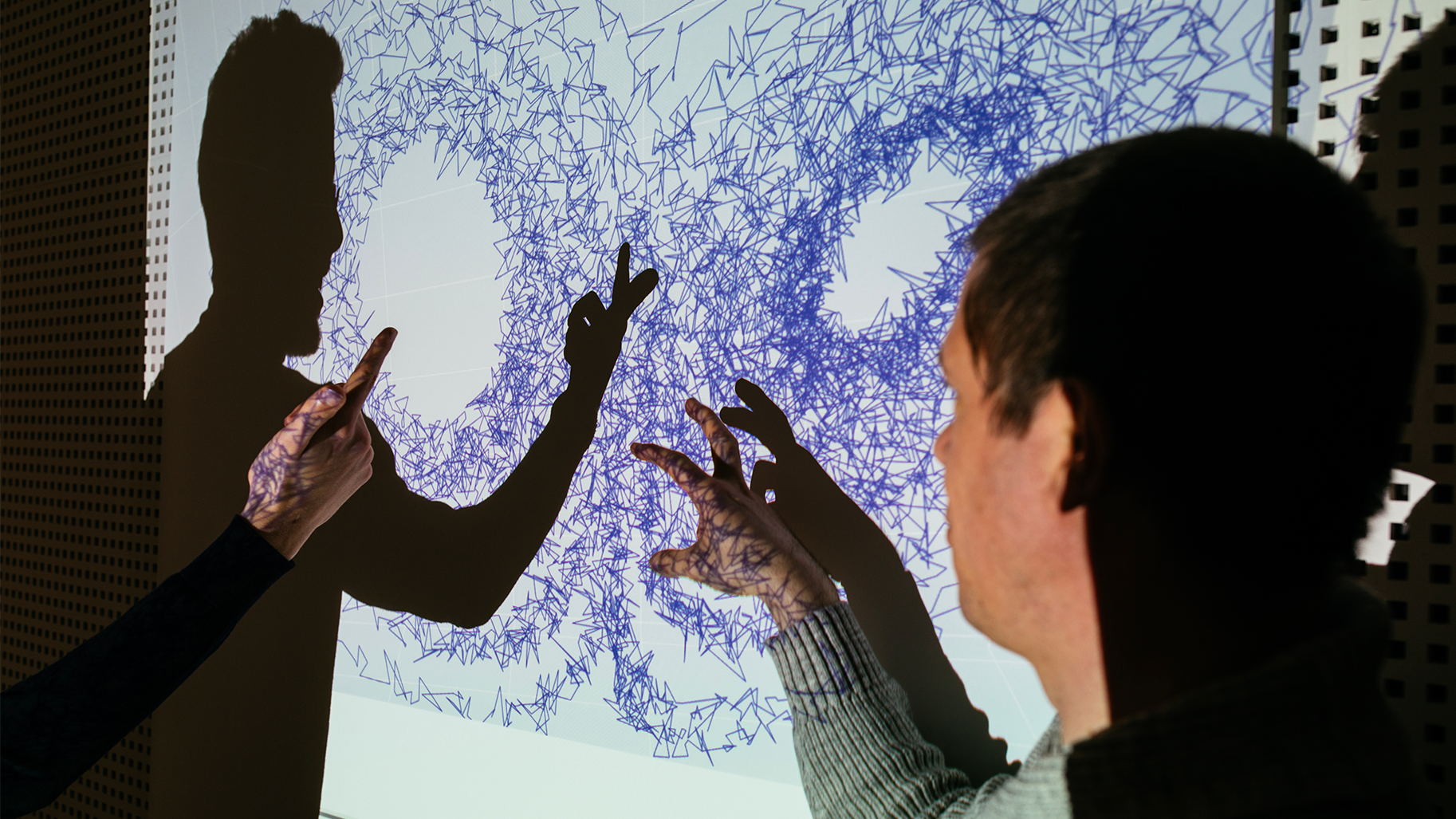
Explore new predictive models
Predicting is not understanding. At least, not yet! The Concaust exploratory project, led by researcher Nicolas Brodu of the Geostat project team, could well lead to the emergence of a new generation of models. Its objective is to explore new predictive tools that focus on simplicity, interpretability of results and reliability. These models are intended to facilitate the analysis of multidisciplinary scientific data.
Generally speaking, the models used for prediction purposes are classified into two categories. The first, called "mechanistic", are based on a precise knowledge of the physical laws that govern a complex environment, such as a forest. The problem is that there are so many different processes in these systems that establishing a simple large-scale model can be too tedious. The latter rely on generic artificial intelligence algorithms, but these generally do not allow us to understand the relationships between parameters that lead to the predictions they make.
Translated with www.DeepL.com/Translator (free version)
Modeling focused on causality
Faced with this observation, scientists are aiming for a new generation of innovative models based on field observations. They allow to exploit these data to extract new knowledge and explain their predictions. This original idea is inspired by theoretical physics methods born in the 1980s based on the concept of "same cause - same consequence". Thus, each time a system is in the same "causal" state, it always evolves according to the same laws of probability.
For example, a forest in a state of drought will always perform less photosynthesis. But to what extent will its activity be affected? What parameters will influence it and how? To answer this question, the exploratory action model examines the relationships between the parameters of past observations. These may be associations of meteorological data, measurements of CO₂ fluxes, etc. The extracted information defines the different possible states of the studied system, here the forest. Thus, by comparing the current state to all states observed in the past, the model predicts the likely futures of our forest.
The objective is not to compete with mechanistic models that will always do better on specific cases. In the same way, machine learning models - using the "like attracts like" principle - are already very useful and efficient to make predictions. On the contrary, Concaust aims at an intermediate way leading to a good "predictability" while keeping a good "interpretability".
Facilitate the interpretability of data
The causal model identifies the information that will explain the dynamics of the system at a given scale. For example, the seasonal cycle influences the annual evolution of photosynthesis, with a maximum in summer and a minimum in winter when the trees are bare. It also depends on the nature of the plants or crops that cover the area to be modeled.
In each case, the Concaust model makes it possible to find the indicators already known by the researchers, such as those mentioned above. But the scientists also hope to find others that were previously underestimated or hidden in the data. "Our model always starts with a problem of interest to the discipline that will use it," explains Nicolas Brodu. For example, Brodu and his collaborator Yao Liu are interested in the parameters involved in the resilience of terrestrial ecosystems. In other words, their capacity to return to a stable state after having been disturbed (parasitic infection, drought, etc.).
Responding to multidisciplinary scientific issues
In order to verify his hypothesis, Nicolas Brodu tested his method on the evolution of the El Niño climatic phenomenon in collaboration with the experts Luc Bourrel and Pedro Rau who have been studying it for years. This phenomenon is punctuated by cyclical oscillations in sea surface temperature and winds. At its extreme, it affects in a reversed way the continental areas on each side of the South Pacific. These areas are sometimes affected by severe droughts and fires, sometimes by heavy rains and devastating floods.
Scientists wondered whether this model would be able to detect the beginnings of rare extreme events sufficiently in advance. The result: the model is able to detect deviations leading to extreme events up to six months in advance on past data. "Since each of these events is different, it is not yet possible to conclude on the occurrence of future events, but these preliminary results are already very encouraging," says the researcher. The model also identifies several key pieces of information to explain these dynamics: the annual cycle of El Niño, the rainfall - both already known - but it also highlights the important role played by the global strength of the oscillations.
"For the moment, the existing indicators are very generic. We would like to assess whether our model can provide more relevant indicators according to each typology of the affected regions (coastal, arid, humid, high altitude, etc.), as they do not have the same constraints," adds the researcher. Such information would make it possible to determine alert thresholds based on data easily measured in the field to warn of the arrival of a major event. The next step is to check whether it works.
What did this exploratory action bring you?
Verbatim
Exploratory action gives us the financial and institutional means to take risks without the demands of publications and immediate results. It has allowed me to do background work that will feed more applicative research for years to come.
Leader of the exploratory action Concaust
Pour aller plus loin
- El Niño – La Niña' oscillations, Concaust
- Inria’s Exploratory Actions: taking risks, Inria, 04/30/2021