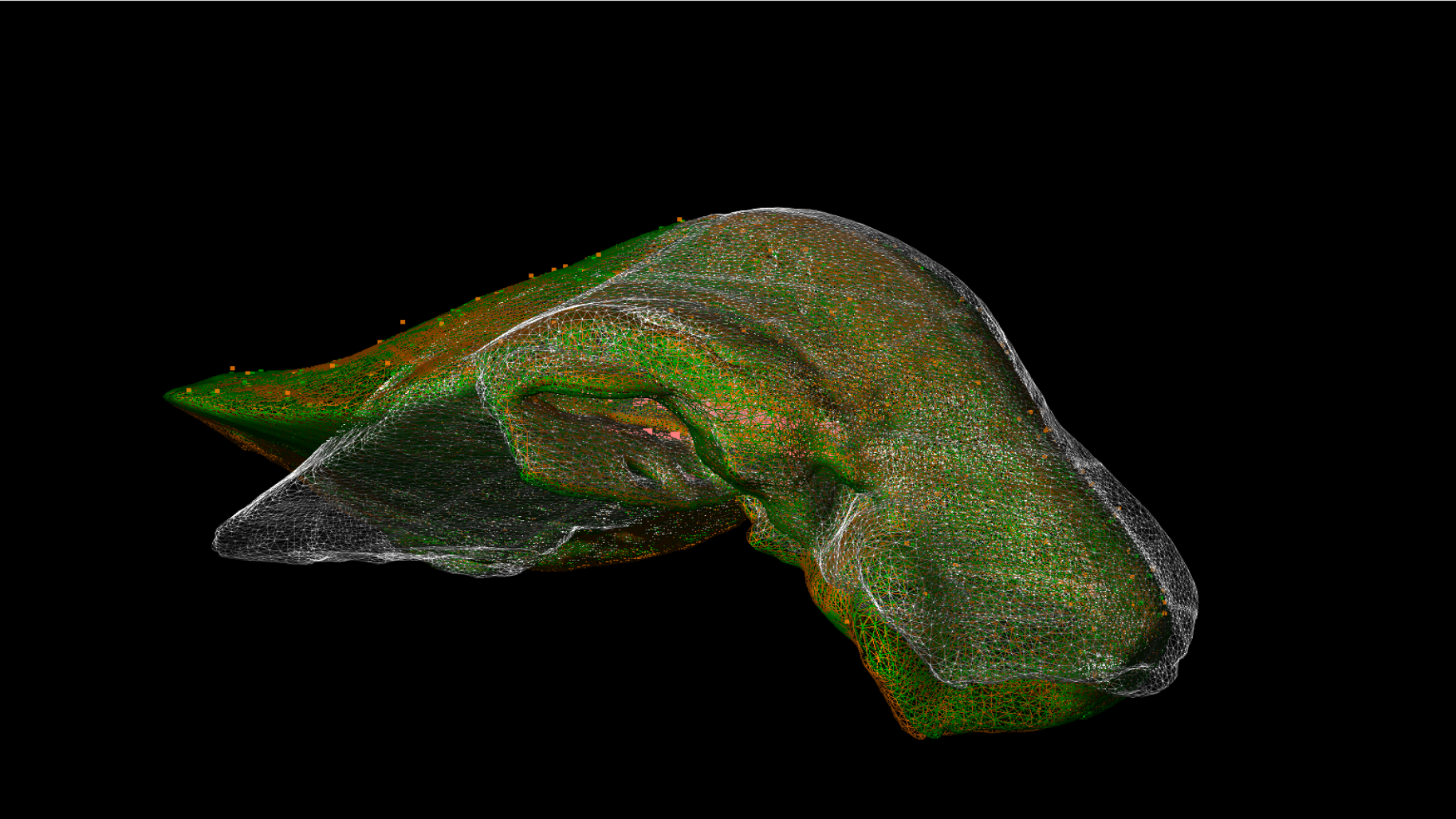
Assisting surgery using numerical simulation
Whether it’s optimising production from a wind turbine, limiting the gas consumption of a generator, assessing the wind resistance of an engineering structure, consolidating weather or climate forecasts, or modelling the propagation of gravitational waves, the use of numerical simulation has become standard in a range of different fields within science and industry. New applications are emerging, particularly in medicine and surgery, where simulation can be used by practitioners to understand and predict the impact of a surgical procedure using mathematical models, in addition to accurately recreating its effects.
In this field, Robin Enjalbert and Alban Odot - the first an engineer and the second a third-year PhD student working within the Mimesis project team at the Inria Nancy – Grand Est research centre - have devised an innovative approach: establishing dialogue between digital technology and artificial intelligence algorithms - including neural networks - in order to improve the performance of simulations. In terms of what applications their research might have, the aim is to enable “real-time” simulations, such as tools to assist surgeons in preparing for or performing operations in theatre.
The advantages of a joint honours degree in science and health
A graduate of Télécom Physique Strasbourg, a non-specialist engineering school, Robin Enjalbert also studied Science and Technology for use in health (robotic surgery, medical imaging, biomechanics, etc.), where he quickly developed an interest in applications involving advanced digital technology. “I was introduced to certain methods used in artificial intelligence during my second-year placement. I was then able to explore the subject in greater detail, focusing on applications in the field of health, during my final year internship at Inria, where I was supervised by Stéphane Cotin, head of the Mimesis project team. A joint honours degree gives you a real advantage when it comes to developing innovations in the field of digital health.”
One of the areas of focus for Mimesis is the use of AI and simulations to assist with certain types of surgical procedure, including fitting catheters. Digital assistants draw chiefly on advanced simulations. “The aim is to develop mechanical models of human organs. For this we use computer science tools with the capacity to recreate the highly complex behaviour of a living being”, explains Alban Odot. “The tissue in an organism, for example, can change shape quite dramatically: digital tools have the capacity to model this ‘hyperelasticity’.”
This is the case for SOFA, an open-source software platform for multiphysics simulation (involving multiple different physical components, such as fluid or structural mechanics, for instance) which Inria first launched more than ten years ago. SOFA now boasts a great many models, solvers and algorithms, enabling the rapid development of new simulations, including those of interest to the medical and health sectors.
The impact of neural networks on simulations
However, simulations require a significant amount of processing time. In order to accurately predict changes to the shape of an eye, the heart or blood vessels, for example, algorithms require multiple “iterations”: they begin by estimating a rough value (accurate to within 10%), before gradually fine-tuning the calculation in order to arrive at the necessary level of precision (accurate to within 1%, 0.1% or potentially even less, as the case may be).
This iterative process is long, with each step in the process taking at least ten seconds: too long for applications in health, where complex changes to the shape of organs have to be predicted in real-time. The aim is to adapt augmented reality tools capable of being used by surgeons during procedures, or to teach certain tasks, such as navigation for endovascular surgery.
But how can this process be accelerated? The Mimesis team employs the use of artificial intelligence, as Alban Odot explains: “Our research is based on the principle of ‘teaching’ neural networks the physics of an object, training them using a wide array of samples of how the shape of this object might change. The data required for learning is produced using SOFA numerical simulations. Once it has been trained, the network will then be capable, when prompted, of predicting changes to the shape of this object within a simulation, with excellent levels of resolution and taking up very little processing time.” The process is highly effective: “Only a few milliseconds are required with this method of prediction, making it far more efficient than the iterative method”, says the researcher.
The versatility of a generic tool
But numerical simulation and artificial intelligence employ the use of different methods, meaning that one of the major challenges for this research is finding a way of getting digital tools to work together effectively. “I have spent more than a year developing DeepPhysX, a development environment which lets you interface machine learning algorithms with numerical simulations”, explains Robin Enjalbert. “Coded using Python, DeepPhysX lets you use synthetic data taken from numerical simulations in order to train neural networks, and then use these same networks as components in these numerical simulations.”
Comprising different elements responsible for managing the flow of data between the “simulation” and “machine learning” components, and other functions for enabling these two elements to interface with each other, DeepPhysX was designed to be compatible with all types of tools (including those which use commercial computer codes for simulations, like in industry). What this means is that DeepPhysX has the potential to access a whole host of development applications and environments. It is already being used by a number of PhD students within Mimesis for different purposes, whether it’s generating machine learning data, training neural networks or applying predictions from trained networks to simulations.
Taking the first steps in an innovative sector
The tool is currently based on one single AI method (the supervised training of networks with an input tensor and an output tensor), but Robin Enjalbert has his sights set on furthering the potential of DeepPhysX: “The aim of future developments will be to attain greater flexibility when it comes to transferring data, paving the way for other AI methods, such as reinforcement learning or graph neural networks.”
In the field of digital health, the first applications of tools are beginning to emerge, including in ocular surgery, which is the area of focus for InSimo, a start-up with its roots in Inria research. With interest in the subject booming, Robin Enjalbert and Alban Odot, who both have a background in advanced technology, will doubtless have further innovations to showcase to the worlds of research and industry in the future. For them, the adventure is only just beginning...